Designing Explainable Mental Health and Well-being Interventions
Designing
efficient, cost-effective, accessible, trustworthy and acceptable.
We designed a qualitative study involving interviews and scenarios to answer our research questions. We took a qualitative approach to gain a deep understanding of issues that may influence the acceptability of recommendations from the perspective of potential users. We presented participants with five hypothetical yet realistic scenarios involving a smartphone app that utilises sensing, which was mocked-up as an interface prototype using Adobe XD (see Figure \ref{fig:scenario}). We interviewed participants while reviewing each scenario using a set of prompts, enabling us to tease out their perceptions and feelings about what was acceptable to them (or not) when reviewing the recommendation. All of our procedures received approval from The University of Melbourne's ethics committee (2056123.1).
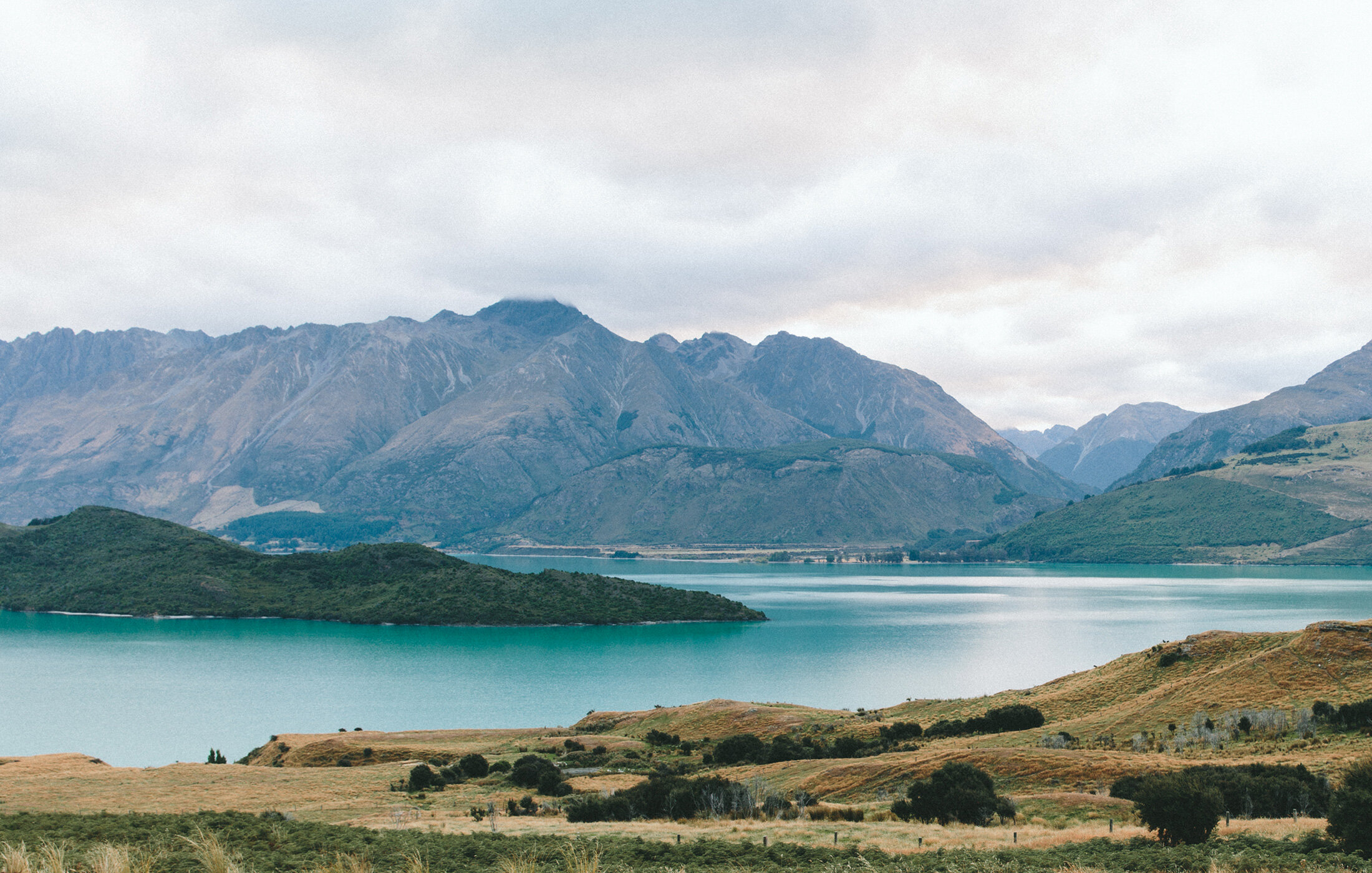
Make it stand out.
It all begins with an idea. Maybe you want to launch a business. Maybe you want to turn a hobby into something more. Or maybe you have a creative project to share with the world. Whatever it is, the way you tell your story online can make all the difference.
Psychologists and clinicians have increasingly advocated using digital technologies to support mental health and well-being.
This has become increasingly important of late, as in-person services have been disrupted or moved to mediated channels due to the COVID-19 pandemic, which has also increased the global demand for mental health services.
Even before the pandemic, there was already an overwhelming shortage of qualified workers—especially in low and middle-income countries.
Hence, we urgently need technology-based solutions that are efficient, cost-effective and accessible but also trustworthy and acceptable.
Hello, and thank you all for tuning in to this presentation. I'll be presenting a paper that examined, and also promotes, explainable recommendations for personal sensing technology acceptance.
This work was conducted during my previous employment at The University of Melbourne, where I was a Research Fellow in Human-Centred Explainable AI.
I am currently affiliated with Lancaster University here in the UK, where I work primarily with research on eye-tracking applications.
This paper was co-authored with Ryan Kelly, Simon D'Alfonso and Reeva Lederman, all of whom work at the intersection of technology and mental health and well-being. Simon is attending the conference virtually and will be happy to answer any questions.
Let's continue on.
The last decade has seen significant interest in the HCI and UbiComp community in developing digital technologies for mental health and well-being.
We have become very good at detecting behaviours through sensor technology and also designing mechanisms for increasing intervention receptivity and adherence.
We have also observed a large increase in smartphone applications that attempts to address mental health from developers.
This paper hopes to bridge these two areas.
This paper focuses on user acceptance, which is one of the primary problems associated with personal sensing.
The focus on user acceptance to date has typically been centred around users' perceptions of being passively tracked and assessed.
While this is important, it is equally important to ensure users also accept the recommendations based on personal sensing.
This is because any design and implementation effort towards sensing-based interventions will ultimately be unsuccessful if users do not accept its outcome.
We use the term "personal sensing" in this paper, which refers to the continuous collection of data from sensors embedded in digital devices such as smartphones and wearable trackers.
This has also been referred to as digital phenotyping, context sensing and mobile sensing, and passive sensing.
There are numerous advantages of using personal sensing for addressing the high demand for mental health and well-being services, which we have summarised in the paper.
This work takes a two-pronged approach by investigating personal sensing acceptance from two perspectives:
First, the key considerations for the acceptability of recommendations based on personal sensing.
—extending previous research that investigated the acceptance of being tracked by sensors.
Our study identified six design issues for enhancing the acceptability of recommendations based on personal sensing.
Second, how the availability of an explanation can affect personal sensing acceptance.
Our study found that the availability of an explanation enabled participants to understand the rationale behind the recommendations better and correct their assumptions while providing them with a sense of agency, which positively influenced their willingness to accept the recommendation based on personal sensing.
To clarify, the focus is on the availability of explanations and how it affects user acceptance, and not on finding out users' preferences between them.
With these findings, this paper contributes a better understanding of the ways in which we can make personal sensing technology more acceptable.
STUDY
[RQs]
To answer both questions, we designed a qualitative study involving interviews and scenarios. We took a qualitative approach to gain a deep understanding of issues that may influence the acceptability of recommendations from the perspective of potential users.
[scenarios]
We presented participants with five hypothetical yet realistic scenarios involving a smartphone app that utilises sensing.
We used the scenarios to make the idea of personal sensing concrete and to help participants imagine themselves in the situation, rather than asking them to respond to abstract ideas about personal sensing technologies that they might not be familiar with.
[example]
These scenarios are plausible scenarios based on prior work. For example, the scenario presented in the paper focuses on difficulty with sleeping, which is an everyday problem that is known to affect well-being and is a common symptom across mental health conditions.
[screenshots]
We mocked up the hypothetical smartphone app using Adobe XD.
[first]
The first showed a notification with the recommendation (in the form of a question) on the smartphone home screen mockup.
[second]
The second screen showed the explanation of why the recommendation was made in the form of an assessment that typically included behavioural markers.
[third]
Finally, the third screen showed a list of sensors, each with an explanation of how it was used to make the assessment that led to the recommendation.
Further design considerations for these layers and ordering can be found in the paper.
[all]
We consider mental health and well-being interventions to be a subset of recommendations; interventions are, in essence, recommendations for health or behaviour change purposes.
[all scenarios]
Each scenario provided recommendations based on data collected by a range of sensors to survey the acceptability of different use cases and to elicit a range of responses.
We interviewed 25 participants while reviewing each scenario using a set of prompts, enabling us to tease out their perceptions and feelings about what was acceptable to them (or not) when reviewing the recommendation.
All sessions were conducted over Zoom, and we also used a slide deck in which we included the context of the scenario and the prompts to guide the study.
More details on our procedure can, of course, be found in the paper.
Based on our study, we found six key design considerations for the acceptability of recommendations based on personal sensing.
User Personalisation discusses the need for the system to know their individual preferences and circumstances, goals or intrinsic motivations and that the recommendations were tailored to their capabilities and background.
Next, we have appropriate phrasing.
In general, systems should avoid using 'clinical' language and terms that may have negative connotations. Instead, the recommendations should adopt phrasing that is lighter and less direct, with a positive tone.
Third, the system should adapt to their inferred emotional and clinical states, previous assessments and past interactions, and context.
These adaptations were expected to create better adherence to the recommendations without burdening the user to personalise every aspect of the system to suit them.
Users' confidence is about how confident participants felt about the quality of the personal sensing system: why the assessment was made, and whether the underlying system worked as it should.
Participants felt that other users had been assessed by the personal sensing system and if there was information to show that they had followed the recommendations and found them to be helpful.
This awareness may help them normalise the experience of having recommended a course of action due to a detected change in their behaviour.
Lastly, the system should provide users with a sense of agency
such as choosing when to take the recommendations, what recommendations to take, and what is sensed, assessed and recommended.
As for RQ2, our overall analysis indicated that having an explanation at each level improved personal sensing acceptance because it
(1) allowed participants to understand why and how the recommendations were made,
(2) enabled participants to correct their assumptions, and
(3) provided participants with a sense of agency.
In the paper, we provide nuanced discussions on the findings in terms of the value of explainable recommendations for personal sensing technology acceptance,
the feasibility of implementing the six key considerations,
and that we as researchers need to shift our view from looking at sensor acceptance to recommendations based on personal sensing.
To conclude, our findings further suggest that people are becoming more accepting of being passively sensed from their devices, indicating that people may eventually become more open to adopting personal sensing technology to support their mental health and well-being.
As mentioned at the beginning of this presentation, this paper contributes a better understanding of the ways in which we can make personal sensing technology more acceptable.